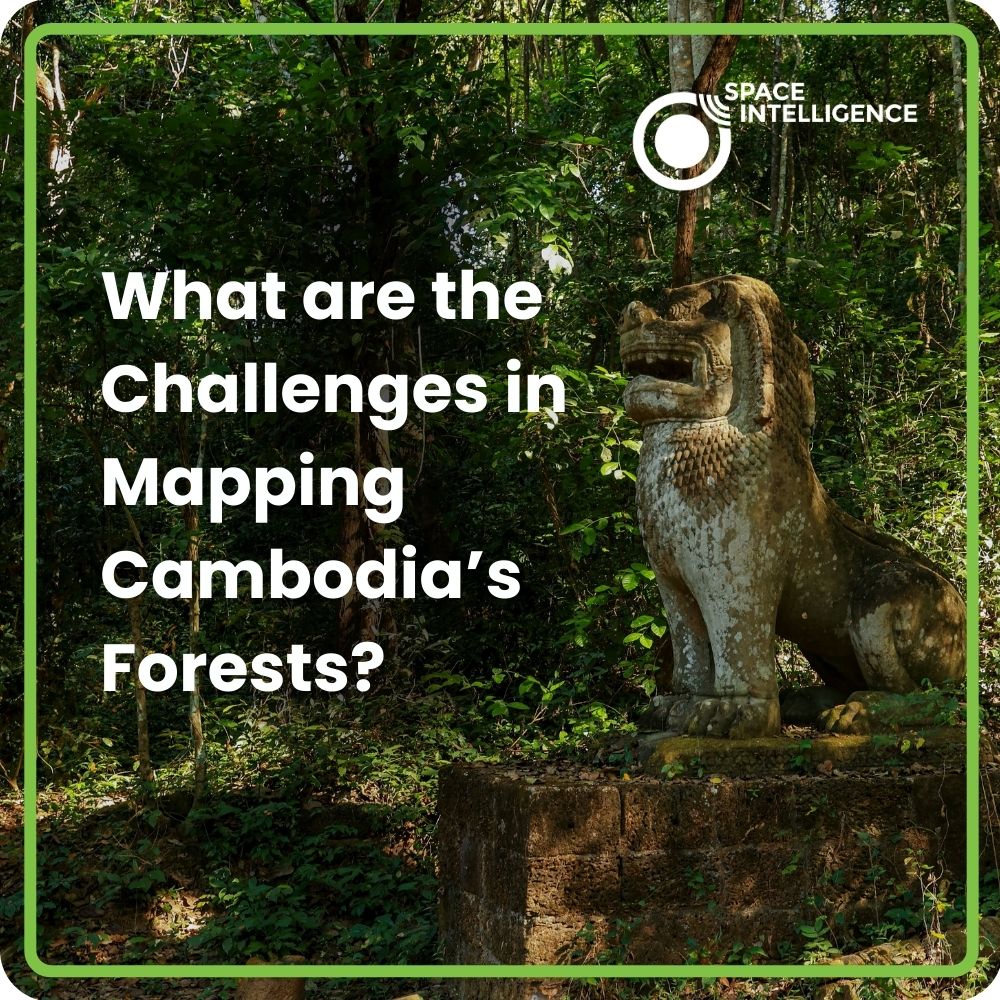
What are the Challenges in Mapping Cambodia’s Forests?
Cambodia hosts a range of forest types including evergreen, semi evergreen, deciduous forests, flooded forests, and mangroves. This is really fantastic from an ecological perspective, but creates challenges in the modelling and the production of reliable land cover change data.
These forests look vastly different at different times of year and so can lead to inaccuracies into statistics like deforestation rates if remote sensing processes aren’t calibrated to these factors.
This was a key element which we had to overcome when producing our land cover maps of over 18 million hectares in Cambodia across multiple time points.
Seasonality’s Impact on Mapping
The below example is a very clear demonstration of the impact of seasonality on forests and remote sensing processes: this is a seasonally dry forest within the same year, and there’s a stark difference in the optical imagery.
These dynamics need to be captured by both the integration of ecological expertise in map creation and sampling, but also through capturing multiple time points across a year. If only using optical data from a single time point to assess this area’s land cover classification, and without a human-in-the-loop validation process, it would likely misclassify the status of the forest.
Dealing with Extreme Weather Events
Another challenge we encountered in mapping Cambodia was that in 2015 there was El Nino event, which very sadly caused widespread droughts across the country and with far reaching consequences for the people of Cambodia. The results were seen across the landscape, spanning agricultural lands and forests.
From a modelling perspective, this presented a challenge: in such droughts, we might expect both changes in forest phenology (the timing of when trees grow and shed their leaves) and tree mortality. The former is still forest but if we observe sufficient amounts of tree loss, this would no longer be a forest.
By mapping multiple time points around 2015 we could make inferences around the viability of forest loss in affected areas as we wouldn’t expect a forest to be cleared and then regrow to similar levels within such a short time period.
What impact do these factors have on deforestation analysis? How did we solve for them?
The Space Intelligence process has been designed to produce high accuracy data across challenging landscapes. Our national land cover maps have been produced with an overall accuracy of >92%, providing end-users with confidence they are making decisions on data that accurately reflects what’s happening on the ground.
Watch our Cambodia Ecosystem Insights webinar on-demand to learn more about how we created our high accuracy national land cover dataset of the country:
https://www.space-intelligence.com/webinar-ecosystem-insights-cambodia/